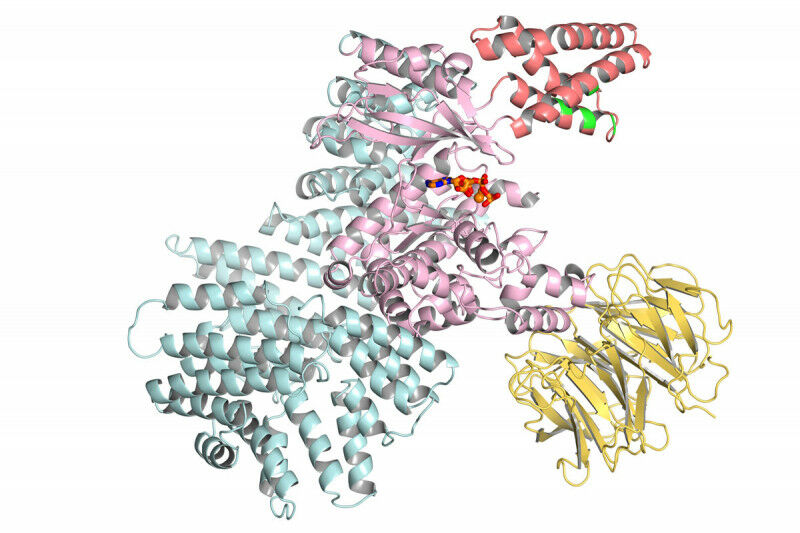
Just one week after Google's DeepMind AI group finally described its biology efforts in detail, the company is releasing a paper that explains how it analyzed nearly every protein encoded in the human genome and predicted its likely three-dimensional structure—a structure that can be critical for understanding disease and designing treatments. In the very near future, all of these structures will be released under a Creative Commons license via the European Bioinformatics Institute, which already hosts a major database of protein structures.
In a press conference associated with the paper's release, DeepMind's Demis Hassabis made clear that the company isn't stopping there. In addition to the work described in the paper, the company will release structural predictions for the genomes of 20 major research organisms, from yeast to fruit flies to mice. In total, the database launch will include roughly 350,000 protein structures.
What’s in a structure?
We just described DeepMind's software last week, so we won't go into much detail here. The effort is an AI-based system trained on the structure of existing proteins that had been determined (often laboriously) through laboratory experiments. The system uses that training, plus information it obtains from families of proteins related by evolution, to predict how a protein's chain of amino acids folds up in three-dimensional space.
The three-dimensional structure that results can give us critical information about the protein, such as how it interacts with other proteins and chemicals and where on the protein chemical reactions occur. Using the structure, researchers can learn how specific mutations, like the ones that cause genetic diseases, alter the protein's function. Researchers can also use the structure to design chemicals that can interact with the protein and change its function, something that has led to therapies for various cancers and HIV.
Normally, these structures are determined by isolating the protein, preparing it for imaging, and bombarding it with electrons. These techniques are difficult and time-consuming, and they often fail. The paper estimates that decades of lab work have left us with structural information for only 17 percent of the full set of human proteins.
That explains why researchers have also spent decades looking for ways to predict structures for proteins using nothing but the sequence of amino acids that make them up. But prior to AlphaFold, the accuracy of software wasn't high enough to be consistently useful.
The human protein collection
DeepMind didn't attempt to predict the structure of every protein in the human genome; some are simply too large to be handled conveniently. (The company set the size cutoff at 2,700 amino acids, which is unfortunately smaller than a gene I spent a chunk of my post-doc cloning.) But most proteins are far smaller than that, so the final count is 98.5 percent of the expected proteins in the genome. Some of these proteins are only predicted to exist based on features of DNA sequences within the human genome.
Just as importantly, AlphaFold includes a confidence estimate that registers how likely its predictions are to be accurate. All told, the software is confident about the location of about 60 percent of the amino acids it has predicted, and it's highly confident about a bit over a third. Put differently, the researchers have a confident prediction about most of the structure of 40 percent of human proteins. Obviously, that means there's a considerable amount of work to do before we can say we have a good grip on the full set of human proteins. But that's still a lot more than the 18 percent we have actual structures for.
There is also a large collection of proteins that aren't well-represented by existing structures. Those embedded in a cell's membrane are difficult to isolate and work with, so researchers haven't solved many structures of these membrane proteins. But despite having fewer examples in its training data, AlphaFold seems to handle the structures reasonably well.
Where does the system run into problems? Many proteins simply don't form a defined structure—in fact, their function seems to depend on having a completely flexible structure in order to function. Obviously, it's hard to make any accurate predictions of a structure here, since these proteins (more typically, sections of proteins) have none. There are also many proteins that only take on their structure when they are in contact with another protein or a chemical. Since AlphaFold doesn't have that information, there is not a lot it can do.
In general, the DeepMind team found that AlphaFold had very low confidence in its predictions for disordered regions, and they could use that information to identify areas of proteins that are likely to be unstructured.
It’s all going public
At some point in the near future (possibly by the time you read this), all this data will be available on a dedicated website hosted by the European Bioinformatics Institute, a European Union-funded organization that describes itself in part as follows: "We make the world’s public biological data freely available to the scientific community via a range of services and tools." The AlphaFold data will be no exception; once the above link is live, anyone can use it to download information on the human protein of their choice.
Or, as mentioned above, the mouse, yeast, or fruit fly version. The 20 organisms that will see their data released are also just a start. DeepMind's Demis Hassabis said that over the next few months, the team will target every gene sequence available in DNA databases. By the time this work is done, over 100 million proteins should have predicted structures. Hassabis wrapped up his part of the announcement by saying, "We think this is the most significant contribution AI has made to science to date." It would be difficult to argue otherwise.
That said, there are still some issues left to be worked out. There will undoubtedly be improvements made to the algorithm with time, so there will need to be a system to handle updating and versioning in the main database. DeepMind has also made the code for AlphaFold open source, so there's the potential for forks and other complications.
But those problems are worries for the future. For now, we can all sit back and watch the servers strain to service nearly every biologist on the planet who is curious to see whether a protein that interests them has a high-quality structure.
(Except your humble author, since my protein of choice was annoyingly oversized.)
Nature, 2021. DOI: 10.1038/s41586-021-03828-1 (About DOIs).
Recommended Comments
There are no comments to display.
Join the conversation
You can post now and register later. If you have an account, sign in now to post with your account.
Note: Your post will require moderator approval before it will be visible.